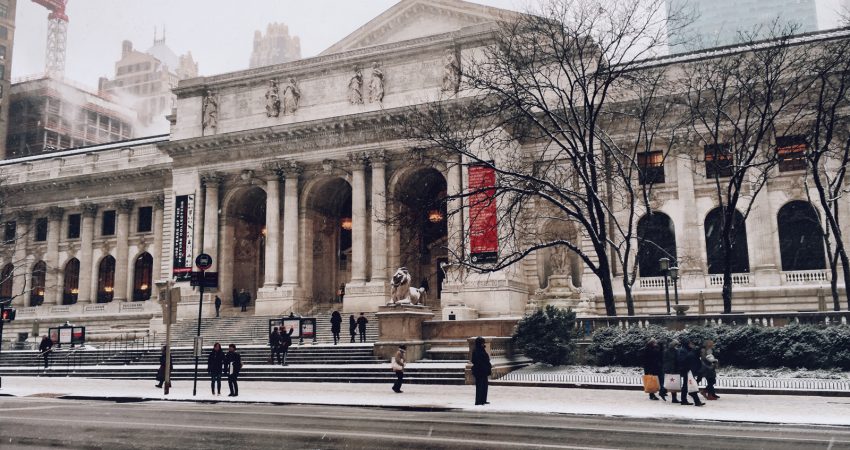
By Elaine Regan - March 2011
PAPER CITATION
Krantz, A., Korn, R., & Menninger, M. (2009). Rethinking museum visitors: Using K-means cluster analysis to explore a museum's audiences. Curator: The Museum Journal, 52(4), 363–374.
This paper presents one quantitative strategy (K-means cluster analysis) for exploring museum-motivated ideas that can be helpful in resource allocation, marketing, event planning, and designing exhibits. Cluster analysis provides an alternative way of knowing and understanding visitors, especially when the rating statements used in the questionnaire and in the analysis represent the museum's intentions.
K-means cluster analysis is a well-accepted exploratory statistical technique in social science research that creates natural, internally similar groups from rating scale questionnaire data. The authors present the adage "birds of a feather flock together" as a means of conceptualising the idea. At the centre of each cluster is the mean of all the data within that cluster, called thecentroid.
Data for cluster analysis are generated from rating scales on questionnaires, which can provide information on visitors' thoughts, attitudes, beliefs, and behaviours in the form of interval data. The quality of the rating scales is paramount to the resulting clusters. Therefore, it is important to consider what the museum wants to know or the question that the museum wants to answer when devising rating statements since these will become the variables in the analysis. Questionnaire design requires careful planning in order to design, test, pilot, and administer a questionnaire (see Oppenheim, 2000). For a cluster analysis, all respondents must rate all of the statements.
Central to conducting K-means cluster analysis is deciding on how many clusters are specified to the algorithm. Usually two, three, or four clusters are generated and reviewed to identify which grouping is most natural. The statistical program identifies the centroid for each cluster by running the algorithm until a stable solution with minimum variability within each cluster and maximum variability between each cluster results. It is at this point that the researcher examines the outputs and applies an interpretation and judgment as to whether the clusters are natural and relevant to the museum.
There are some criticisms of this method relating to its lack of rigor and robustness, but it is a well-accepted exploratory technique. Understanding how clusters are most similar and dissimilar is of particular benefit to ISE staff who may wish to examine the clusters to determine aspects of a program that will be least successful in reaching a particular cluster, or determine how to better focus a program to particular characteristics of a cluster. Some application examples are presented in the paper.
Survey research can be insightful, but it requires specialized skills to design, pilot, administer, analyze, and interpret the data. This may not be available internally within the informal context and may prove costly to outsource.
For futher reading, see
Oppenheim. A. N. (2000). Questionnaire design, interviewing and attitude measurement.Continuum International Publishing Group Ltd. London.